Maximize Your Machine Learning Projects with an Image Annotation Tool for Machine Learning
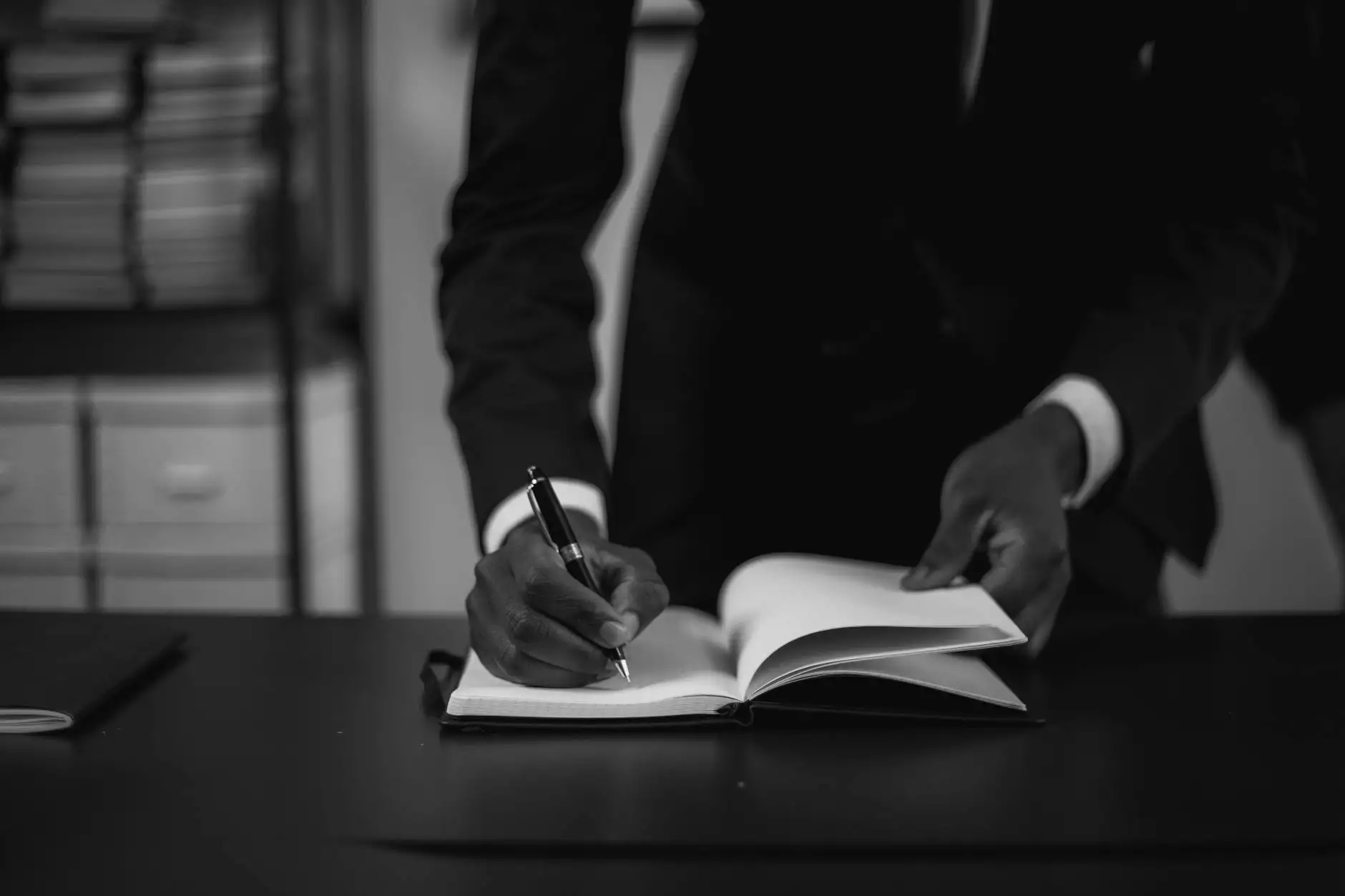
In the rapidly evolving world of artificial intelligence, the importance of efficient data management cannot be overstated. A critical component of any machine learning (ML) project is the quality of the data used to train the models. This is where an image annotation tool for machine learning comes into play, acting as a crucial facilitator in the process of labeling images for computer vision tasks.
Understanding Image Annotation and Its Significance in Machine Learning
Image annotation is the process of identifying and labeling objects within an image. It serves as the fundamental step in creating datasets for training machine learning models, particularly in the field of computer vision. By employing an image annotation tool, businesses can streamline this process, ensuring that their machine learning models are trained on high-quality, accurately labeled data.
Why Is Image Annotation Essential?
The significance of image annotation cannot be overlooked. Here are some key reasons:
- Enhanced Model Accuracy: Accurate annotations lead to better model predictions, as the machine learns to identify features within the labeled data.
- Efficient Training: Properly annotated data accelerates the training process, allowing models to learn faster and more effectively.
- Improved Usability: Annotated datasets can easily be shared among teams and organizations, fostering collaboration and innovation.
- Scalability: With an image annotation tool, businesses can scale their data labeling processes to meet the demands of larger projects holistically.
The Process of Image Annotation
Understanding the workflow of image annotation can help businesses leverage the right tools for their projects. The standard process typically includes the following steps:
1. Data Collection
Gather the images needed for your machine learning project. This could include photographs, screenshots, or any images relevant to your application.
2. Selection of the Annotation Tool
Choose an image annotation tool for machine learning that fits your project’s needs. Popular tools include:
- Labelbox: Known for its user-friendly interface and integration capabilities.
- VGG Image Annotator: A free tool widely used in academic circles.
- RectLabel: A robust option for Mac users focusing on image segmentation.
3. Annotation Tasks
Using the chosen annotation tool, start labeling your images. Common tasks include object detection, image segmentation, and image classification. Each task serves a different purpose, and choosing the right one depends on your specific use case.
4. Quality Assurance
After labeling, it’s crucial to verify the accuracy of the annotations. This might involve cross-checking with other team members or using automated quality control tools incorporated into advanced annotation software.
5. Dataset Export
Once verified, export the annotated dataset in the required format. Most machine learning frameworks require specific formats, so ensure compatibility for a smooth training process.
Choosing the Right Image Annotation Tool
With numerous options available, selecting the right image annotation tool is paramount to the success of your machine learning project. Consider the following factors:
1. User Interface
A user-friendly interface can significantly reduce the time spent on training team members to use the tool effectively.
2. Annotation Features
Different tools offer various annotation capabilities. Choose one that supports the specific types of annotations you need, such as bounding boxes, polygons, or keypoints.
3. Collaboration Features
If your team is working remotely or across different locations, ensure the tool supports collaboration features, enabling multiple users to work on the project simultaneously.
4. Integration Capabilities
Choose a tool that easily integrates with your existing workflows and machine learning platforms.
5. Cost
The budget is always a concern. Evaluate whether the tool provides value for its cost based on your specific project requirements.
Benefits of Using an Image Annotation Tool for Machine Learning
Implementing an image annotation tool for machine learning can significantly enhance the efficiency and effectiveness of your ML projects. Here are some pivotal benefits:
1. Automation
Many modern annotation tools offer automated features to speed up the labeling process, saving both time and human resources.
2. Enhanced Collaboration
With tools designed for teamwork, teams can review, comment, and track changes in real-time, improving project management and communication.
3. Historical Data Tracking
Most annotation tools keep track of changes over time, allowing teams to revisit previous iterations of the dataset and understand how annotations have shifted.
4. Scalability
As the quantity of data grows, many tools allow teams to efficiently scale their annotation processes without sacrificing quality.
Challenges in Image Annotation
While using image annotation tools provides numerous advantages, challenges do still exist:
1. Ambiguity in Annotation Guidelines
Inconsistent guidelines can lead to varying interpretations among annotators, resulting in poor-quality annotations. Clearly defined guidelines are essential.
2. Resource Intensive
Data labeling can be time-consuming and require significant human resources, especially if handled manually.
3. Quality Control
Ensuring the quality of annotations can prove challenging. Establishing a procedure for reviewing annotations is crucial to maintain accuracy.
Conclusion
In the realm of machine learning, the integration of a robust image annotation tool for machine learning is essential for developing accurate and effective models. As businesses continue to recognize the value of high-quality data, the choice of annotation tool becomes increasingly important. By understanding the processes involved and knowing the benefits and challenges associated with image annotation, organizations can make informed decisions that drive their machine learning initiatives forward.
Investing in the right tools not only enhances the quality of your datasets but also streamlines your workflows and accelerates project timelines. Remember, in the world of AI, the data holds the key, and proper annotation is your gateway to unlocking its potential.