Unlocking the Potential of Bounding Boxes in Data Annotation Tools
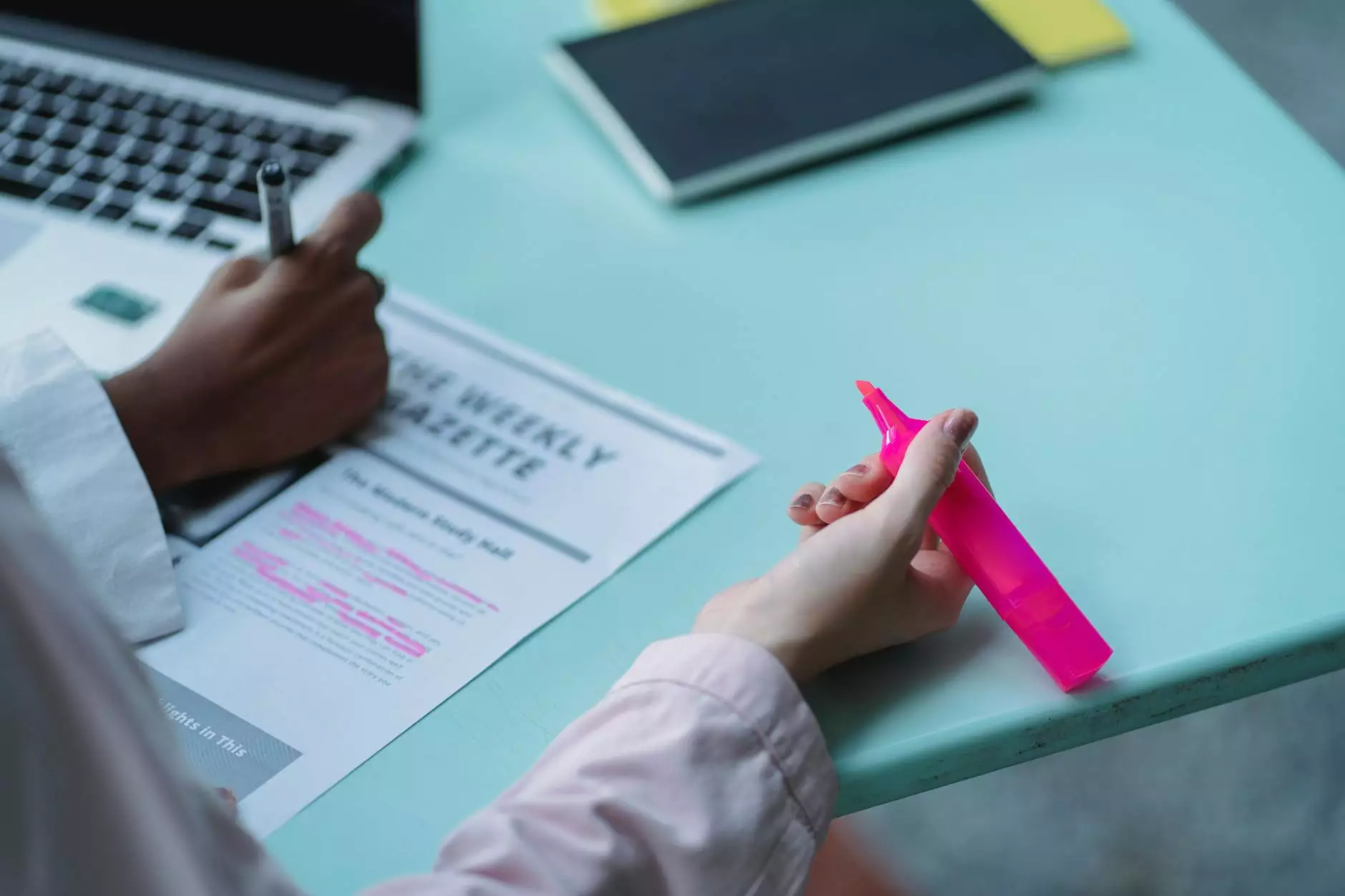
In today's increasingly digital world, businesses are constantly on the lookout for innovative solutions to maximize their data utilization. One such crucial component of data annotation tools is the concept of bounding boxes. Understanding bounding boxes and their application can significantly enhance the efficiency of data annotation and revolutionize how companies operate in the fields of machine learning and artificial intelligence.
What Are Bounding Boxes?
Bounding boxes are rectangular boxes used primarily in image processing and computer vision tasks. They are defined by coordinates that specify the top-left and bottom-right corners of the rectangle, effectively enclosing a particular object within an image. This visual demarcation allows machine learning algorithms to identify and locate instances of the labeled objects accurately.
The Importance of Bounding Boxes in Data Annotation
In the realm of data annotation platforms, the usage of bounding boxes is paramount. Here's why:
- Facilitating Object Detection:Bounding boxes enable precise object detection. When an AI model is trained using labeled images where objects are identified by bounding boxes, it learns to recognize the shapes, sizes, and positions of these objects within different contexts.
- Enhancing Accuracy: The utilization of bounding boxes sharpens the accuracy of machine learning models. Clear annotations lead to better model training, resulting in improved performance when deployed in real-world scenarios.
- Efficiency in Annotation: Using bounding boxes simplifies the annotation process for human annotators, expediting the speed at which large datasets can be prepared.
The Application of Bounding Boxes in Various Sectors
Bounding boxes are extensively utilized across diverse industries. Understanding their applications can provide a clearer picture of their significance:
1. Autonomous Vehicles
In the automotive industry, particularly in the development of autonomous vehicles, bounding boxes are vital for object detection systems. These systems need to identify pedestrians, other vehicles, road signs, and obstacles in real-time. By accurately labeling objects with bounding boxes, developers ensure that the navigation systems of these vehicles can react appropriately to their surroundings.
2. Medical Imaging
In healthcare, bounding boxes are used to label specific areas or abnormalities in medical images, such as X-rays or MRIs. This annotation assists in training AI models to recognize diseases or conditions, contributing to faster and more accurate diagnostics.
3. Retail and Inventory Management
In the retail sector, bounding boxes help in inventory management systems by allowing businesses to automatically identify and track products on shelves through image recognition technologies. This reduces human error and streamlines inventory processes.
Implementing Bounding Boxes: Best Practices for Data Annotation
When utilizing bounding boxes for your data annotation needs, consider the following best practices:
- Consistency: Maintain a standardized approach to drawing bounding boxes across your dataset. This includes size, shape, and placement to ensure uniformity.
- Detailed Guidelines: Create comprehensive guidelines for annotators detailing what constitutes a bounding box for different objects. This helps reduce ambiguity and enhances the overall quality of annotations.
- Quality Control: Implement a quality control process to review annotations. This can involve routine checks or using multiple annotators to review the same images to ensure accuracy.
The Technology Behind Bounding Boxes
The effective use of bounding boxes is largely dependent on the technology and tools used in the data annotation process. Below are some key technological aspects:
1. Annotation Tools
Numerous data annotation tools are available that simplify the process of creating bounding boxes. These tools often come with user-friendly interfaces that allow annotators to draw boxes intuitively. Popular tools include:
- LabelImg
- CVAT (Computer Vision Annotation Tool)
- Roboflow
- VGG Image Annotator
2. Integration with Machine Learning Models
The integration of bounding boxes with advanced machine learning models, such as Convolutional Neural Networks (CNNs), enhances the training process. These models require precise labeled data to improve their predictive capabilities, making the role of accurate bounding box annotations essential.
Challenges in Bounding Box Annotation
While the use of bounding boxes is beneficial, it is not without challenges. Some common issues include:
- Ambiguity in Object Boundaries: Sometimes, the edges of objects may not be clearly defined, leading to inconsistent bounding box placements.
- Scaling Issues: In images with deep objects or varying scales, annotators may struggle to accurately create bounding boxes that encapsulate the desired areas.
- Time-Consuming Process: Comprehensive annotations can be labor-intensive, requiring substantial time investment to ensure accuracy and quality.
Future Trends in Bounding Box Annotation
The future of bounding boxes in data annotation platforms looks promising with advancements in technology. Here are some expected trends:
1. Automation and AI-Assisted Annotation
With the rise in AI capabilities, we anticipate a shift towards automated bounding box creation. AI models will increasingly assist human annotators by suggesting box placements based on pre-trained patterns, significantly reducing the time required for manual annotation.
2. Enhanced User Interfaces
As demand for data annotation grows, tools will evolve, offering more intuitive user interfaces that allow for easier creation and modification of bounding boxes. Enhanced interfaces will likely incorporate drag-and-drop features and smart suggestions based on previous annotations.
3. Integration with Augmented Reality (AR)
As AR technologies advance, we might see systems that utilize real-time bounding box generation for training AI models directly from live video feeds, further enriching the data available for model training.
Conclusion: Embracing Bounding Boxes for Business Success
Bounding boxes are more than just rectangles on images; they possess significant implications for business success in the realms of data annotation and AI development. As we advance into an era focused on intelligent applications, understanding and implementing bounding boxes effectively will be pivotal for organizations striving to harness the full potential of their data. The adoption of high-quality bounding boxes in data annotation tools is not just a technical necessity but a strategic business advantage.