Unlocking Efficiency: The Ultimate Guide to Picture Annotation Tools
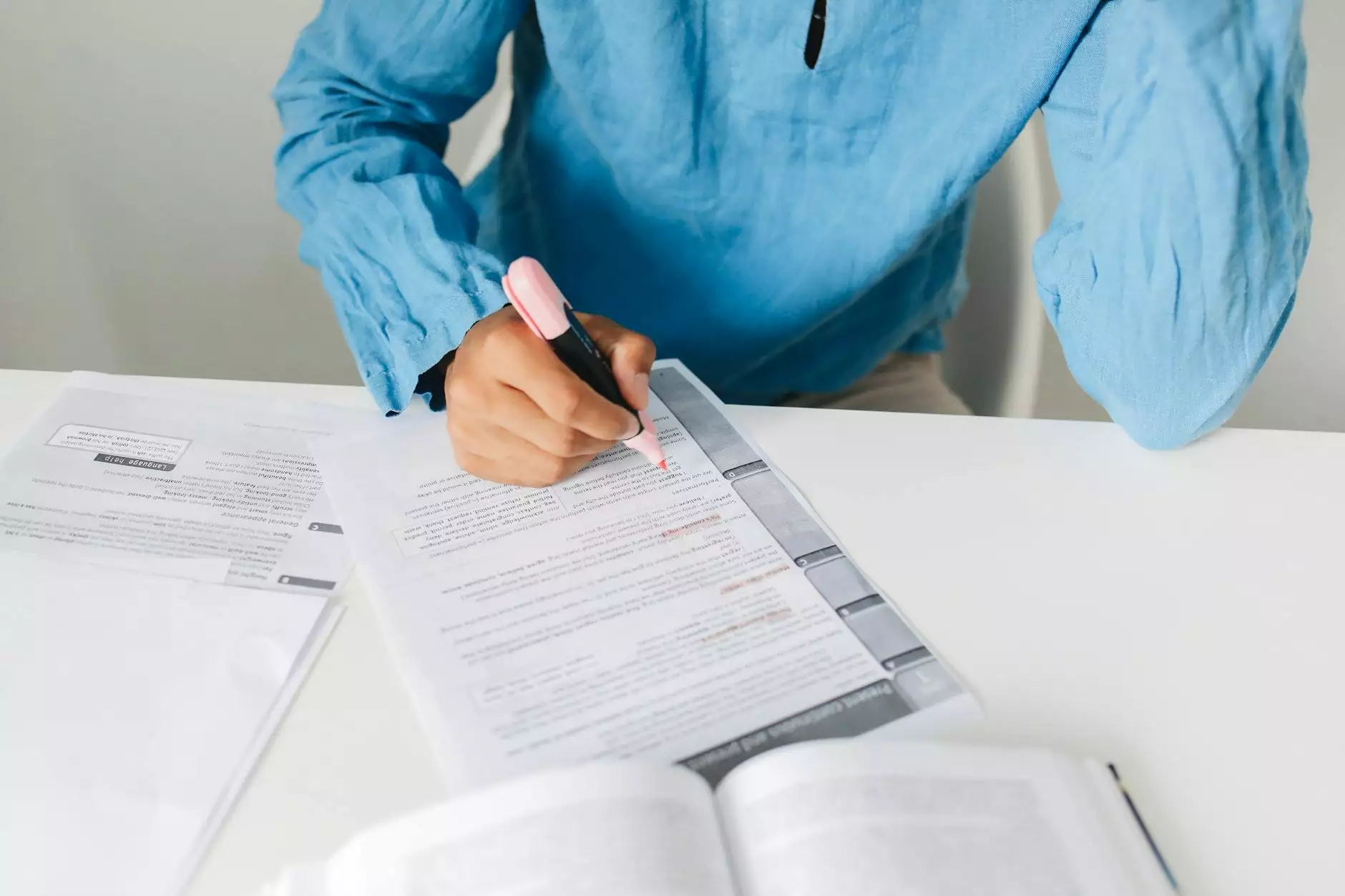
In the age of artificial intelligence and machine learning, the significance of annotated data has escalated dramatically. Businesses across various sectors are increasingly relying on high-quality data to develop and enhance their AI models. One of the key components of this process is the use of picture annotation tools, which play a pivotal role in training AI algorithms by providing them with precisely labeled datasets.
What is Picture Annotation?
Picture annotation refers to the process of labeling images with relevant information to create training data for computer vision applications. This process can involve various forms of tagging, including:
- Object Detection: Identifying and locating objects in an image.
- Image Segmentation: Dividing an image into segments to identify specific areas or objects.
- Bounding Box Annotation: Drawing rectangles around objects to signal their location.
- Semantic Segmentation: Assigning a semantic label to every pixel in the image.
- Keypoint Annotation: Identifying specific points on an object, such as facial features.
The Importance of Picture Annotation Tools for Businesses
Businesses are leveraging picture annotation tools to streamline their data preparation processes, ensuring that they have high-quality datasets for training their machine learning models. Here's why these tools are essential:
- Enhanced Accuracy: Accurate annotations lead to better trained models, which in turn result in reliable predictions and classifications.
- Time Efficiency: Automating the annotation process with advanced tools can drastically reduce the time required to prepare datasets.
- Scalability: Picture annotation tools offer scalable solutions that can cater to various project sizes, from small datasets to large-scale operations.
- Collaboration: These tools often provide collaborative features allowing teams to work together seamlessly.
Key Features of Effective Picture Annotation Tools
When selecting a picture annotation tool, it is crucial to look for specific features that can significantly enhance your experience:
- User-Friendly Interface: A simple and intuitive interface ensures quick adoption and minimal training time.
- Multi-Format Support: The ability to annotate various image formats (JPEG, PNG, etc.) and integrate with existing workflows is vital.
- Advanced Annotation Types: Choose tools that support various annotation features such as polygon, line, and subdivision annotations.
- Quality Control Mechanisms: Tools that provide options for reviewing and validating annotations contribute to higher accuracy.
- Integration Capabilities: Compatibility with other tools or platforms, such as machine learning frameworks, can streamline processes.
- Automation Features: Utilizing AI to assist in the annotation process can substantially reduce manual work and speed up the workflow.
Recommended Picture Annotation Tools
Several tools are recognized as leaders in the market for data annotation, each with unique features catering to different business needs. Here are some top contenders:
- Labelbox: A powerful platform that supports numerous annotation types and integrates seamlessly with machine learning pipelines, providing a collaborative environment for teams.
- SuperAnnotate: Known for its AI-powered features, SuperAnnotate facilitates accurate image segmentations and object detections while ensuring a user-friendly experience.
- Scale AI: This tool offers high-quality annotations at scale and is geared towards enterprises looking for a reliable data annotation partner.
- Keylabs: A frontrunner in the annotation space, Keylabs.ai offers an intuitive data annotation platform that accelerates the process of creating high-quality labeled datasets.
Best Practices for Using Picture Annotation Tools
To maximize the benefits of your chosen picture annotation tool, consider implementing these best practices:
1. Define Clear Annotation Guidelines
Establishing comprehensive guidelines for annotators will help ensure consistency across your dataset. This includes:
- Defining what constitutes each label clearly.
- Providing examples of correct annotations.
- Outlining the process for handling ambiguous cases.
2. Utilize Automation Where Possible
Take advantage of features that allow automation within your annotation tool. While human judgment is critical, AI assistance can handle repetitive tasks, freeing up your team for more complex decisions.
3. Regularly Review and Validate Annotations
Implement a robust quality control system to periodically review annotations. This step is essential to maintain high quality in your datasets.
4. Encourage Team Collaboration
Utilizing tools with strong collaboration features can greatly enhance your team's efficiency. Foster communication and allow team members to share feedback on annotations in real-time.
5. Continuously Train Your Annotators
Investing in ongoing training for your team keeps them updated on the latest best practices, tool features, and industry trends, which can lead to higher quality annotations.
Conclusion
The role of picture annotation tools in the realm of data annotation cannot be overstated. As businesses continue to harness the power of AI and machine learning, having access to high-quality annotated datasets is vital for success. By providing tools that enhance collaboration, streamline workflows, and ensure accuracy, organizations can gain a competitive edge.
Choosing the right picture annotation tool tailored to your specific needs, like those mentioned from Keylabs, will allow you to effectively unlock the potential of your data. As you look to the future, ensure that your data preparation processes are both efficient and accurate to fuel the growth and innovation of your business.
Further Resources
For more information on enhancing your data annotation processes, consider exploring the following resources:
- Keylabs Blog: Insights on data annotation best practices.
- AI Annotation Tips: Tips and tricks for better annotations.
- Data Quality Resources: Ensuring high quality in your datasets.